Several people asked me to dive a bit deeper into large language model (LLM) jargon and explain some of the more technical terms we nowadays take for granted. This includes references to "encoder-style" and "decoder-style" LLMs. What do these terms mean?
Let's get to it: What are the differences between encoder- and decoder-based language transformers?
Encoder- And Decoder-Style Transformers
Fundamentally, both encoder- and decoder-style architectures use the same self-attention layers to encode word tokens. However, the main difference is that encoders are designed to learn embeddings that can be used for various predictive modeling tasks such as classification. In contrast, decoders are designed to generate new texts, for example, answering user queries.
The original transformer
The original transformer architecture (Attention Is All You Need, 2017), which was developed for English-to-French and English-to-German language translation, utilized both an encoder and a decoder, as illustrated in the figure below.
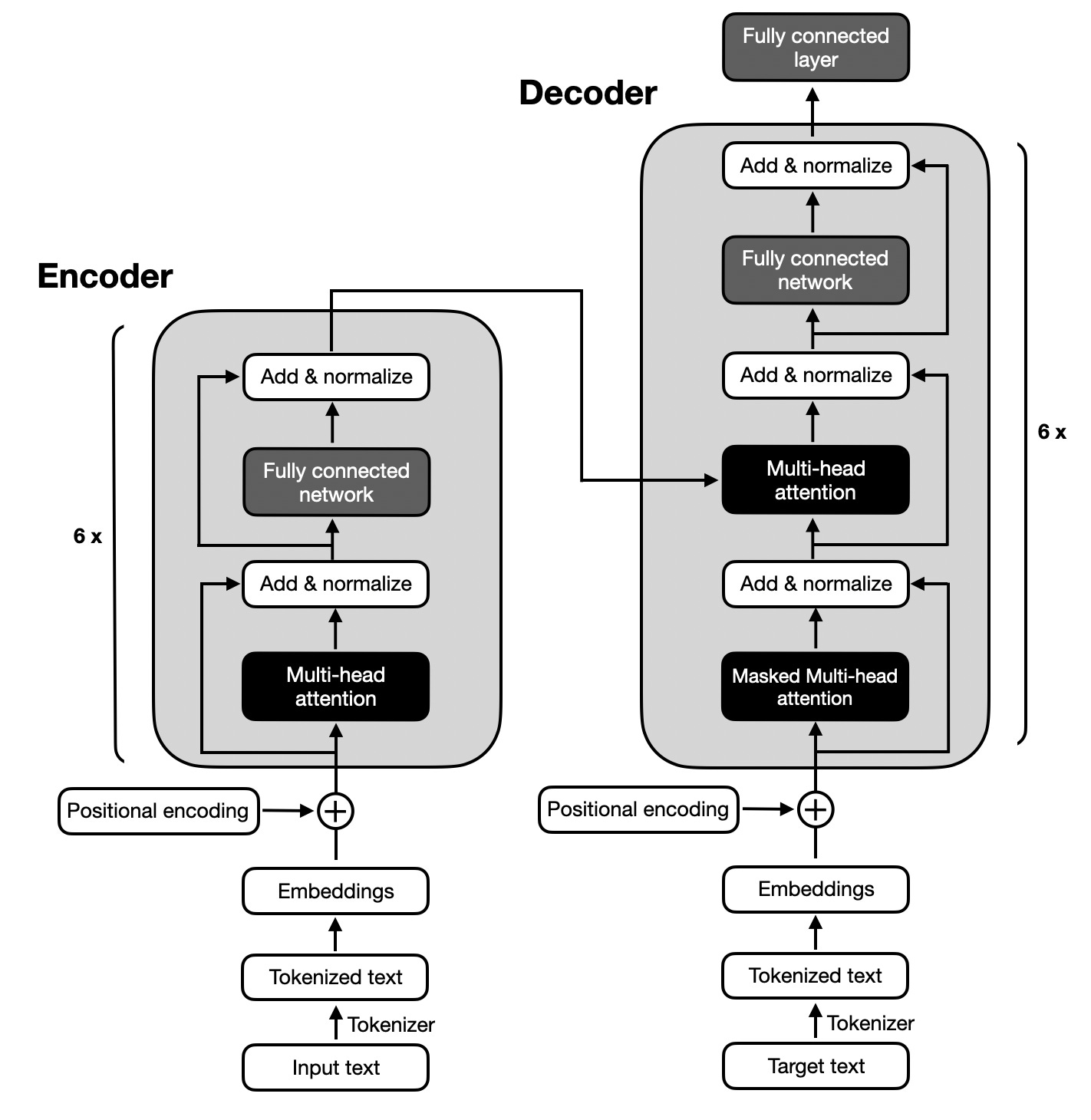
In the figure above, the input text (that is, the sentences of the text that is to be translated) is first tokenized into individual word tokens, which are then encoded via an embedding layer before it enters the encoder part. Then, after adding a positional encoding vector to each embedded word, the embeddings go through a multi-head self-attention layer. The multi-head attention layer is followed by an "Add & normalize" step, which performs a layer normalization and adds the original embeddings via a skip connection (also known as a residual or shortcut connection). Finally, after entering a "fully connected layer," which is a small multilayer perceptron consisting of two fully connected layers with a nonlinear activation function in between, the outputs are again added and normalized before they are passed to a multi-head self-attention layer of the decoder part.
The decoder part in the figure above has a similar overall structure as the encoder part. The key difference is that the inputs and outputs are different. The encoder receives the input text that is to be translated, and the decoder generates the translated text.
Encoders
The encoder part in the original transformer, illustrated in the preceding figure, is responsible for understanding and extracting the relevant information from the input text. It then outputs a continuous representation (embedding) of the input text that is passed to the decoder. Finally, the decoder generates the translated text (target language) based on the continuous representation received from the encoder.
Over the years, various encoder-only architectures have been developed based on the encoder module of the original transformer model outlined above. Notable examples include BERT (Pre-training of Deep Bidirectional Transformers for Language Understanding, 2018) and RoBERTa (A Robustly Optimized BERT Pretraining Approach, 2018).
BERT (Bidirectional Encoder Representations from Transformers) is an encoder-only architecture based on the Transformer's encoder module. The BERT model is pretrained on a large text corpus using masked language modeling (illustrated in the figure below) and next-sentence prediction tasks.
The main idea behind masked language modeling is to mask (or replace) random word tokens in the input sequence and then train the model to predict the original masked tokens based on the surrounding context.
Next to the masked language modeling pretraining task illustrated in the figure above, the next-sentence prediction task asks the model to predict whether the original document's sentence order of two randomly shuffled sentences is correct. For example, two sentences, in random order, are separated by the [SEP] token:
[CLS] Toast is a simple yet delicious food [SEP] It’s often served with butter, jam, or honey.
[CLS] It’s often served with butter, jam, or honey. [SEP] Toast is a simple yet delicious food.
The [CLS] token is a placeholder token for the model, prompting the model to return a True or False label indicating whether the sentences are in the correct order or not.
The masked language and next-sentence pretraining objectives (which are a form of self-supervised learning, as discussed in Chapter 2) allow BERT to learn rich contextual representations of the input texts, which can then be finetuned for various downstream tasks like sentiment analysis, question-answering, and named entity recognition.
RoBERTa (Robustly optimized BERT approach) is an optimized version of BERT. It maintains the same overall architecture as BERT but employs several training and optimization improvements, such as larger batch sizes, more training data, and eliminating the next-sentence prediction task. These changes resulted in RoBERTa achieving better performance on various natural language understanding tasks than BERT.
Decoders
Coming back to the original transformer architecture outlined at the beginning of this section, the multi-head self-attention mechanism in the decoder is similar to the one in the encoder, but it is masked to prevent the model from attending to future positions, ensuring that the predictions for position i can depend only on the known outputs at positions less than i. As illustrated in the figure below, the decoder generates the output word by word.
This masking (shown explicitly in the figure above, although it happens internally in the decoder's multi-head self-attention mechanism) is essential to maintain the autoregressive property of the transformer model during training and inference. The autoregressive property ensures that the model generates output tokens one at a time and uses previously generated tokens as context for generating the next word token.
Over the years, researchers have built upon the original encoder-decoder transformer architecture and developed several decoder-only models that have proven to be highly effective in various natural language processing tasks. The most notable models include the GPT family.
The GPT (Generative Pre-trained Transformer) series are decoder-only models pretrained on large-scale unsupervised text data and finetuned for specific tasks such as text classification, sentiment analysis, question-answering, and summarization. The GPT models, including GPT-2, (GPT-3 Language Models are Few-Shot Learners, 2020), and the more recent GPT-4, have shown remarkable performance in various benchmarks and are currently the most popular architecture for natural language processing.
One of the most notable aspects of GPT models is their emergent properties. Emergent properties refer to the abilities and skills that a model develops due to its next-word prediction pretraining. Even though these models were only taught to predict the next word, the pretrained models are capable of text summarization, translation, question answering, classification, and more. Furthermore, these models can perform new tasks without updating the model parameters via in-context learning, which is discussed in more detail in Chapter 18.
Encoder-decoder hybrids
Next to the traditional encoder and decoder architectures, there have been advancements in the development of new encoder-decoder models that leverage the strengths of both components. These models often incorporate novel techniques, pre-training objectives, or architectural modifications to enhance their performance in various natural language processing tasks. Some notable examples of these new encoder-decoder models include
Encoder-decoder models are typically used for natural language processing tasks that involve understanding input sequences and generating output sequences, often with different lengths and structures. They are particularly good at tasks where there is a complex mapping between the input and output sequences and where it is crucial to capture the relationships between the elements in both sequences. Some common use cases for encoder-decoder models include text translation and summarization.
Terminology and jargon
All of these methods, encoder-only, decoder-only, and encoder-decoder models, are sequence-to-sequence models (often abbreviated as seq2seq). Note that while we refer to BERT-style methods as encoder-only, the description encoder-only may be misleading since these methods also decode the embeddings into output tokens or text during pretraining.
In other words, both encoder-only and decoder-only architectures are "decoding." However, the encoder-only architectures, in contrast to decoder-only and encoder-decoder architectures, are not decoding in an autoregressive fashion. Autoregressive decoding refers to generating output sequences one token at a time, conditioning each token on the previously generated tokens. Encoder-only models do not generate coherent output sequences in this manner. Instead, they focus on understanding the input text and producing task-specific outputs, such as labels or token predictions.
Conclusion
In brief, encoder-style models are popular for learning embeddings used in classification tasks, encoder-decoder-style models are used in generative tasks where the output heavily relies on the input (for example, translation and summarization), and decoder-only models are used for other types of generative tasks including Q&A. Since the first transformer architecture emerged, hundreds of encoder-only, decoder-only, and encoder-decoder hybrids have been developed, as summarized in the figure below.
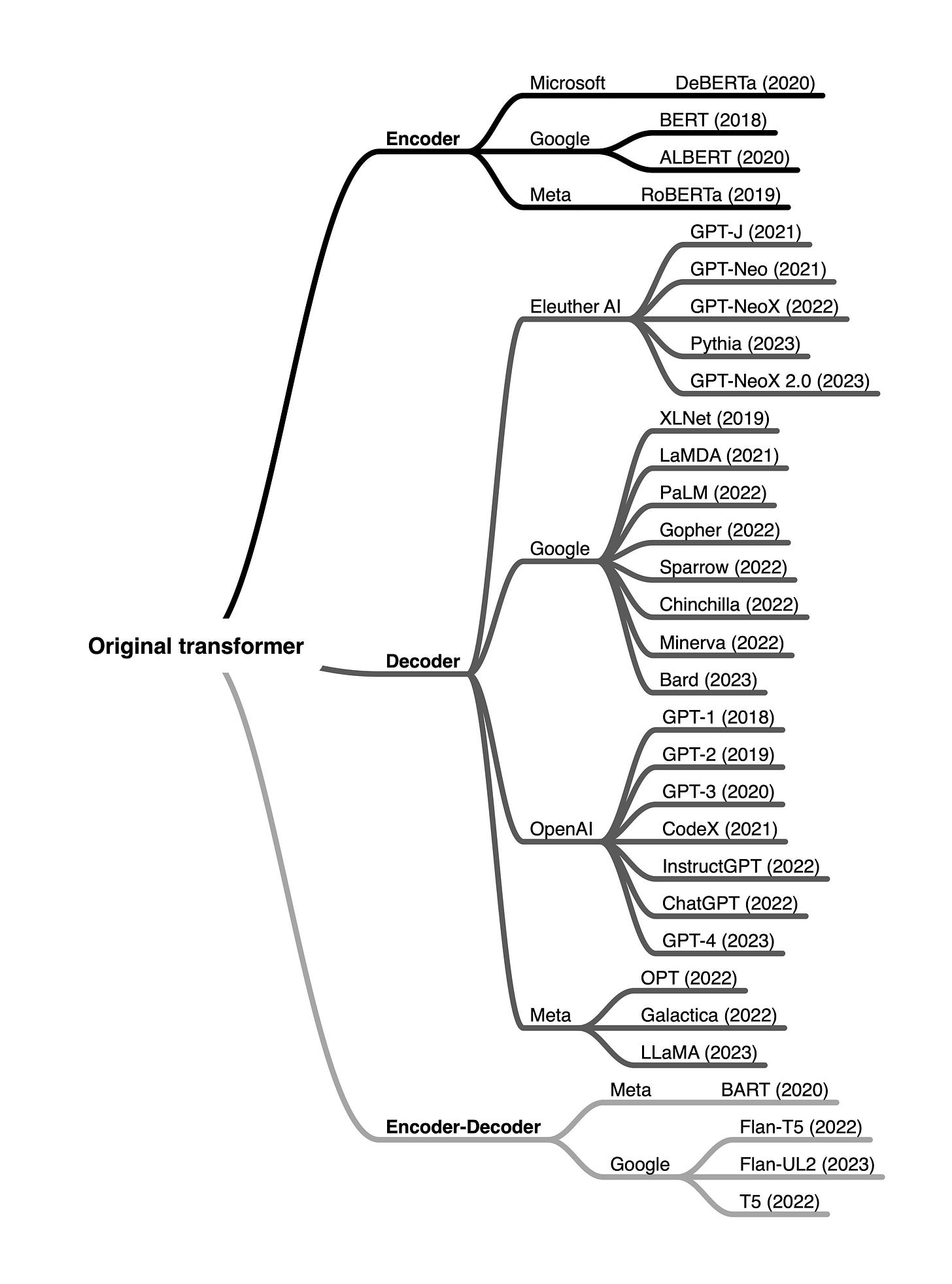
While encoder-only models gradually lost in popularity, decoder-only models like GPT exploded in popularity thanks to breakthrough in text generation via GPT-3, ChatGPT, and GPT-4. However, encoder-only models are still very useful for training predictive models based on text embeddings versus generating texts.
This magazine is a personal passion project that does not offer direct compensation. However, for those who wish to support me, please consider purchasing a copy of one of my books. If you find them insightful and beneficial, please feel free to recommend them to your friends and colleagues.
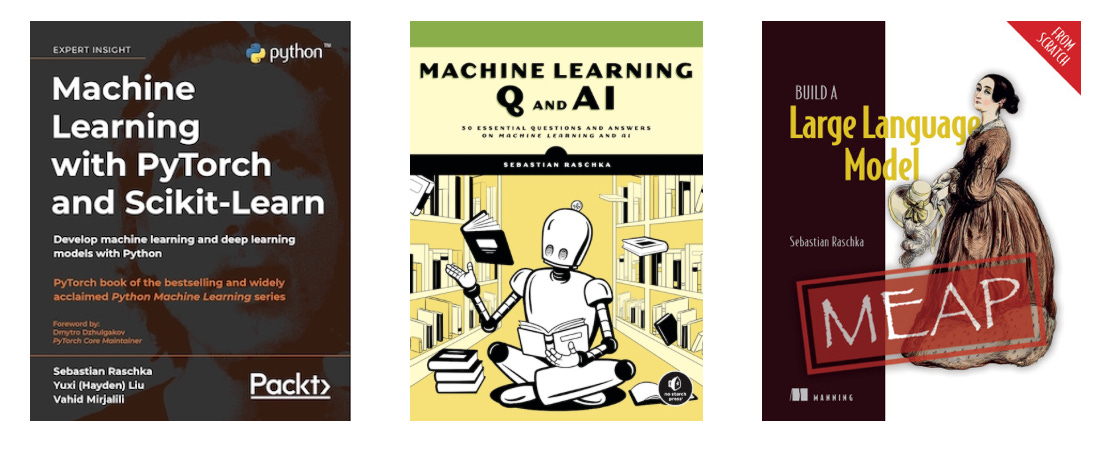
Your support means a great deal! Thank you!
I agree the term encoder / decoder is overloaded since almost all architectures would essentially perform the encoding / decoding as a function. Engineers are not good at naming not only vars after all 🤣
This LLM encoder/decoder stuff messes with my mind! There is something fundamental here that I'm not getting. HELP... 🤔 I have been fascinated with autoencoders, which take an example from feature space and ENCODE it into point in latent space and then DECODE it back into a reconstructed example in feature space, thus allowing a reconstruction loss to be calculated. [ref: Python ML 3Ed, Chap 17]
1) Should LLM decoders be called 'generators' like in GANs?
2) That single line that connects LLM encoder to its decoder... Is that the same data that one receives as an embedding from the LLM API?
3) For a decoder-only LLM, is its input always an embedding vector? Or, where do the model weights come from?
4) Is it possible to take an LLM embedding, reconstruct its initial input, and calculate the reconstruction loss? If true, this would enable us to map the fine (manifold) structures in these mysterious LLM latent spaces. Loved your old examples of putting/removing smiles on celebrity faces. Like to find a few hallucinations lurking in LLM latent spaces! 😮